HRDA: Context-Aware High-Resolution Domain-Adaptive Semantic Segmentation
HRDA: Context-Aware High-Resolution Domain-Adaptive Semantic Segmentation
Basic Information
- Lukas Hoyer, Dengxin Dai, Luc Van Gool @ ETH Zurich & MPI for Informatics
- 2022 ECCV
問題描述
這篇 paper 如同 DAFormer 關注在 UDA for semantic segmentation 。
在過去的方法上由於 UDA 會需要儲存許多不同 Domain 的資訊、額外的 network 架構(如 Knowledge Distilation 時的 Student 與 Teacher),以及各種新定義的 Loss 資訊,導致 GPU 用量普遍很高,也因此在圖片的輸入上通常會刻意先將輸入圖片的解析度降低。
像是 Cityscapes 的圖片解析度有 2048x1024,但是實作時卻使用 1024x512 的大小。
這種 Low-Resolution(LR) 的方法對於辨識細節相當不利,因為無法好好擷取特徵。然而若直接使用高解析度的圖片,即便 GPU 的記憶體空間足夠,也會因為擷取到過於細節的特徵導致大的物件無法好好辨認。
Info
這就像是不同地方的人行道也許會有不同的地磚設計,但我們都會認為那就是人行道。如果我們看過於細節,導致我們對於不同的設計誤以為是不同類型的物件,那就會在辨識上出現問題。
HRDA 的目標是希望結合 Low-Resolution(LR) 能辨識大範圍特徵的優點,以及 High-Resolution(HR) 能辨識細節的優點,創造出在兩個狀況下都能夠順利辨認的方法。
最後,他們提出的 HRDA 方法甚至大幅超越了過去他們提出的 DAFormer。
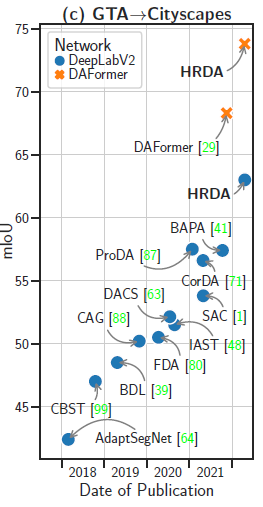
Related Works
- Sematic Image Segmentation
- Unsupervised Domain Adaptation (UDA)
- Self-training
- DAFormer
Methodology
Preliminary
UDA 以 self-training 方法處理的大架構如下圖所示,由於過去的研究當中發現 Knowledge-Distillation 會對於 UDA 有正向的幫助,因此我們會訓練出一個 Student Network,依照 Source Label 以及 Target Pseudo-Label 去訓練,而 Teacher Network 則透過 Exponential Moving Average(EMA) 去更新。
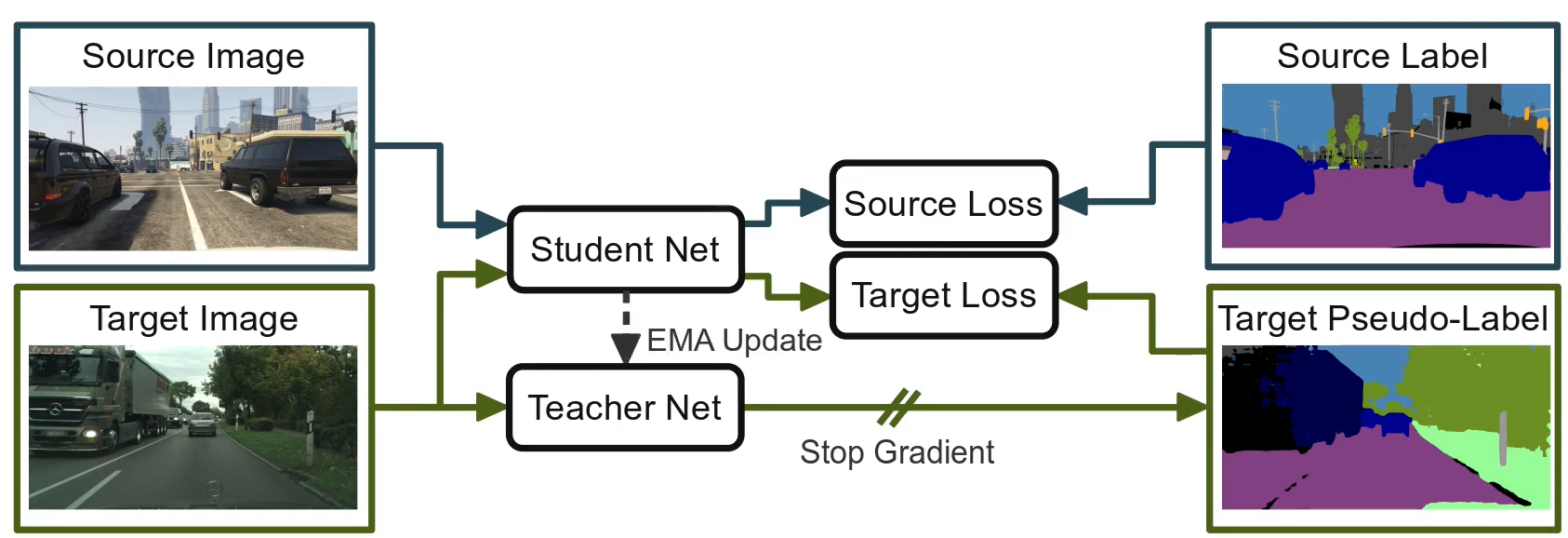
Image from Lukas Hoyer's YouTube Channel
這裡先定義一下接下來會用到的基本 Notation。
- 表示要訓練的模型
- 表示 Source Domain 的資料數量
- 表示 Target Domain 的資料數量
- 表示 Source Domain 的資料
- 表示 Target Domain 的資料
- 表示 Source Domain 對應的 labels
過去的 UDA 方法通常會將輸入的圖片經過一個 bilinearly downsampling function 。例如說 。
經過 可以預測出 source label ,也就可以用 Categorical Cross-Entropy (CCE) 去計算出 Loss。
不過只運用這樣的 Loss 去訓練基本上不會得到太好的結果,過去的經驗上都還需要額外設計一些 Loss function 去幫助訓練。HRDA 採用了 DAFormer 的方法。
關於 DAFormer 可以參考過去的文章
DAFormer 透過 teacher model 去 adapt target domain,透過 可以得到每個 label 預測的機率,我們會從其中取最大的當成是最終的 label 。
Info
這裡的 是 Iverson Bracket,只是單純符合條件給 1
,否則給 0
的符號。
而 student network 就可以透過 pseudo label 去學習 target domain 的資訊。
這裡的 是 DAFormer 當中的信心水平。
如同 DAFormer,在實作上會包含:
- Transformer Network
- Rare Class Sampling
- Thing-Class ImageNet Feature Distance
- Learning Rate Warmup for UDA
Overview
HRDA 做的事情簡單來說就是「透過同時考慮 Low-Resolution 以及 High-Resolution 提升模型的預測能力,且提出技巧避免 GPU 記憶體過量」。整體的架構如下圖。
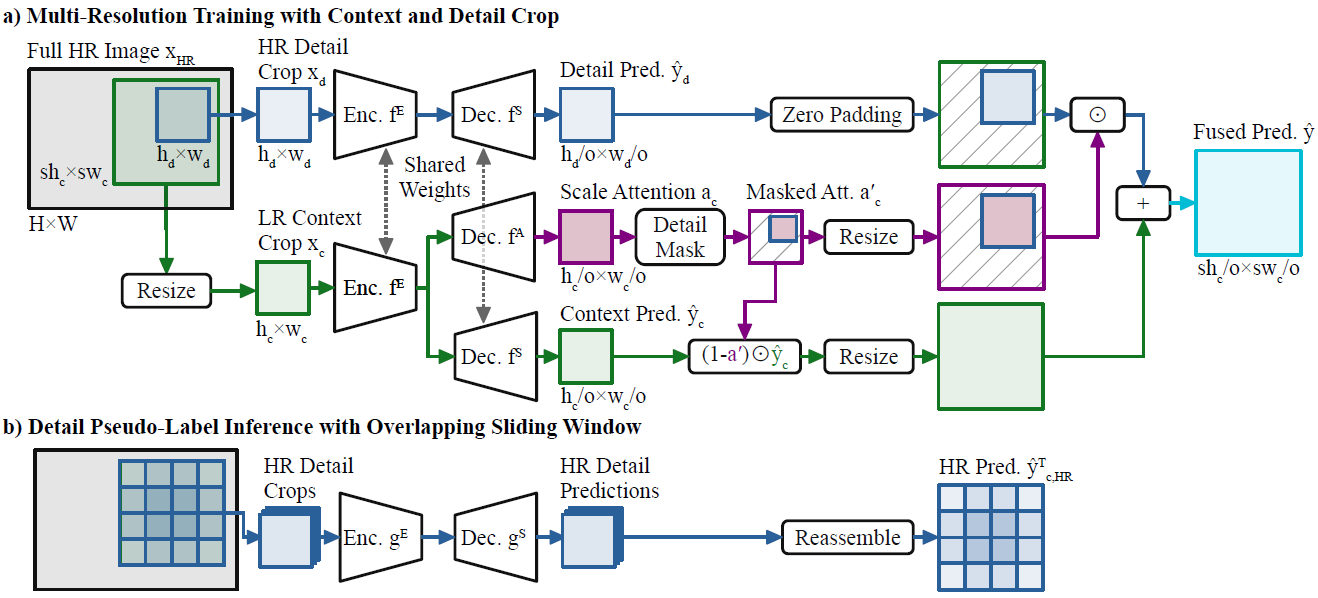
作者在 YouTube 上的簡短說明當中給出的架構圖也許會有更加直觀的理解。
- 將圖片切成 Low-Resolution(LR) 與 High-Resolution(HR) 兩個部分
- 透過 Segmentation Head 得出 LR 與 HR 的預測結果
- 透過 Attention 混合 LR 與 HR 的優勢,並預測出結果
- Fuse 以上的結果,變成最後的預測
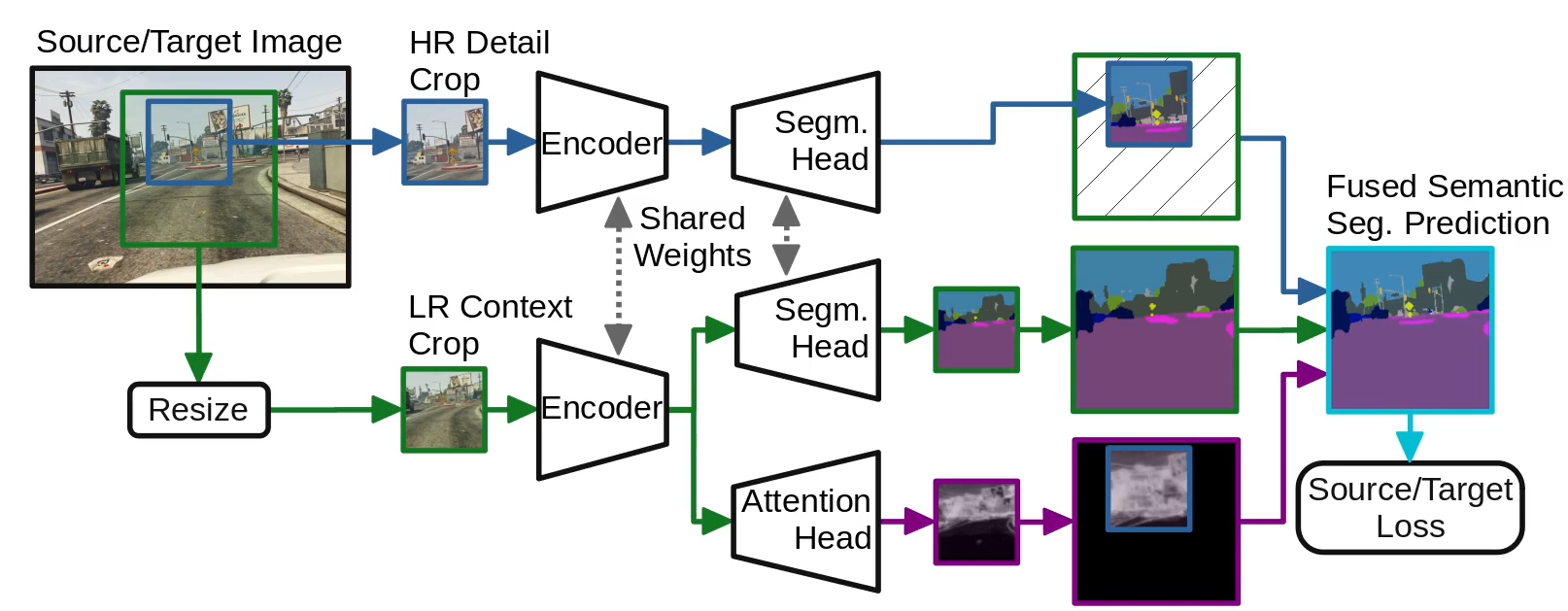
Image from Lukas Hoyer's YouTube Channel
Context and Detail Crop
為了同時保有 Low-Resolution(LR) 能辨識大範圍特徵的優點,以及 High-Resolution(HR) 能辨識細節的優點,HRDA 使用 large LR context crop 以及 small HR detail crop 來分別處理這兩個部分。
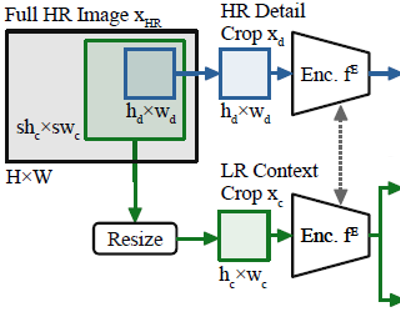
大概念上,對於原本 的圖片,我們想要從中切出一塊 Low-Resolution 的大圖片(Context Crop)、一塊 High-Resolution 的小圖片(Detail Crop)。
HR 會從 LR 當中切出來。更細節來說,LR 的邊長都會是 HR 的兩倍,。
large LR context crop
首先將圖片經過裁切出一部分
接著透過 將裁切後的圖片 downsample 得到 Low-Resolution Context Crop 。
這裡的 用來描述裁切的 bounding box,在選擇上會是在圖片範圍當中的 normal distribution,並且選擇了一個 ( 表示 output stride) 保證選出來的座標可以被 整除,藉此確保後續的運作正常。
small HR detail crop
將 Low-Resolution Context Crop 再進一步裁切得到 High-Resolution Detail Crop 。
其中
Get Semantic Segmentation
接下來透過 Feature Encoder Network 以及 Semantic Decoder Network 就可以分別得到 Context Semantic Segmentation 以及 Detail Semantic Segmentation。
- Context Semantic Segmentation
- Detail Semantic Segmentation
Multi-Resolution Fusion
如同前面所描述,我們知道 LR 與 HR 各有其好處,作者除了分開兩個部分去產出 LR 與 HR 的結果以外,也希望能夠有一個部分能夠讓模型能同時考慮 LR 與 HR 兩者去作出判斷。
從 Low-Resolution 得到大範圍的資訊、從 High-Resolution 得到細節的資訊。再結合 Scale Attention,透過 Attention 機制去判斷現在應該要注重 LR 還是 HR,又分別要給多少的注意力。
於是我們再加上一個 Scale Attention Decoder 去預測 attention scale 來表示一個點要偏向用 LR 或是 HR。
其中 會限制輸出在 之間,越靠近 則越相信 LR,反之則越相信 HR。
接下來,由於 Detail Crop(HR) 只有 Context Crop(LR) 的一部分,所以沒有 HR 的地方只能參考 LR,Attention 設為 。
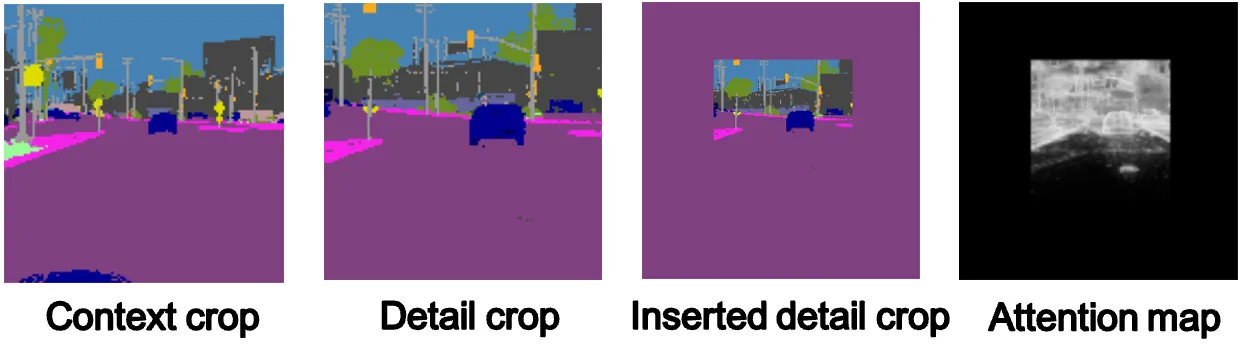
Image from YuKai Chen @ Medium
就像是這邊的 Attention Map。在 Detail Crop 以外的範圍都是黑的,也就是 Attention 為 ,中間的部分才會有 Detail 與 Context 的交互考量。
寫成數學式也許比較難理解,但他只是希望把多的地方設為 而已。
最後要把結果 fuse 在一起,所以要讓大小都相等,對 Detail Crop(HR) 外面補 ,變成跟 Context Crop(LR) 相同。
Attention 可以告訴我們要考慮多少的 Context 以及多少的 Detail,於是把每個點跟 Attention 的值相乘,得到 Context 與 Detail 的結果後相加就會是 Fused 之後的預測結果。
別忘了 Attention 越靠近 則越相信 Context,反之則越相信 Detail
訓練時就會使用 Fused 的版本去訓練,Source 採用的 Loss 是單純的 Cross Entropy 如下。額外考慮 Detail Crop 可以讓模型學習到更好的高解析度特徵。
Target Domain 亦然。不過使用的目標是 pseudo labels,且包含了 quality 的項。
Pseudo-Label Generation with Overlapping Sliding Window
預測的結果並不是每一個點都會考慮到 Detail Crop 的資訊,然而我們會希望每一個地方都能夠精確地考慮,因此會希望能夠取得所有點的 Detail Crop 資訊。
直覺上會覺得,既然我們想要得到全部 Detail Crop 的資訊,那就直接把原圖交給 Network 去處理即可,尤其預測的過程當中並不包含 backpropagation,也就不會有需要儲存過多資訊導致的記憶體不足問題。
然而由於 HRDA 是 based on DAFormer 做出來的,當中包含了 Transformer 架構,會隱晦地在訓練過程中學習到 positional embedding 資訊,因此當訓練時與預測時採用的解析度大小相同會是最理想的。
因此作者採用相同解析度大小,再使用 Sliding Window 去看過整體的圖片,重疊的部分以平均作為結果,給出更穩健的 pseudo label 預測。

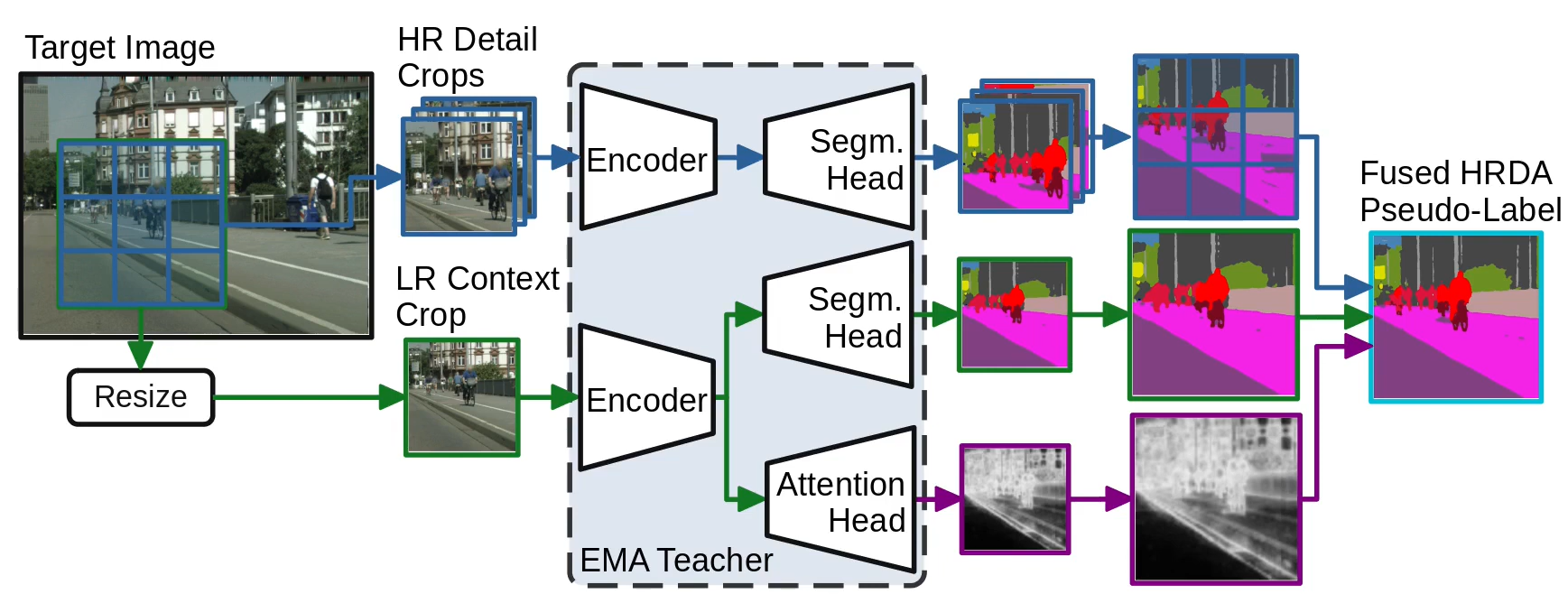
Image from Lukas Hoyer's YouTube Channel
Results
實驗設定
一如既往,這裡使用的 Datasets 包含了 Cityscapes, GTA5, SYNTHIA。
在 Network 的架構上採用 DAFormer,當中包含了 MiT-B5 encoder 以及 DAFormer 中提出的 decoder。在 Scale Attention 的部份使用的是 SegFormer MLP decoder。
在部分與其他 Model 比較的部份為了公平有時會將 DAFormer 的 backbone 換成 ResNet101 或是 DeepLabV2,底下使用不同架構時會再特別提醒。
Overview
與過去的 SOTA 比較的結果如下圖所示。
- 在 GTA5 Cityscapes 當中全部的 Class 都獲得比過去 SOTA 更好的結果,最後的 mIoU 與 DAFormer 相比多了 5.5 的成長。
- 在 Synthia Cityscapes 當中則對大多數的 Class 而言都獲得更好的結果,並且 mIoU 與 DAFormer 相比多了 4.9 的成長。
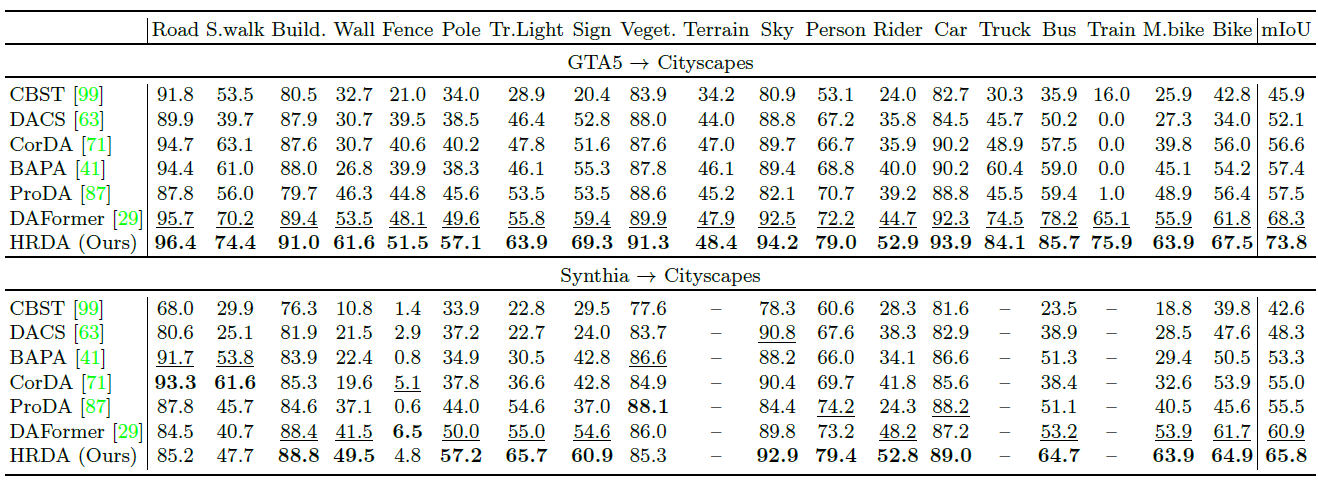
從圖片上來觀察,可以發現到 HRDA 在細節上的處理比起過去的 SOTA 有更好的處理(如:紅綠燈、騎士),並且對於大的物件也同樣保有更好的結果(如:巴士、汽車)。
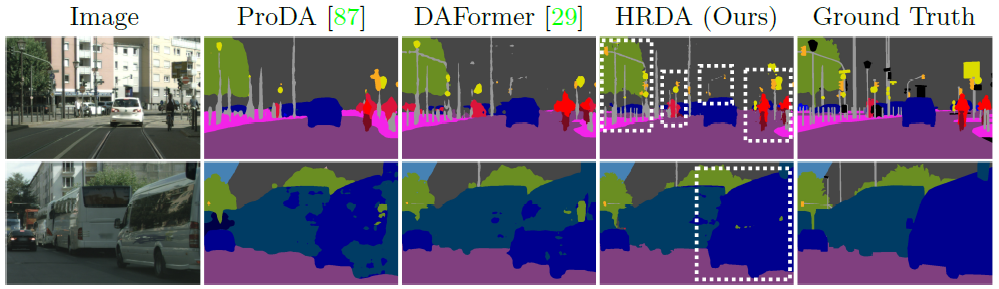
並且如果將 HRDA 應用在過去各種 UDA 的方法上,也可以看出 HRDA 都能夠有效地提升最後的 performance。
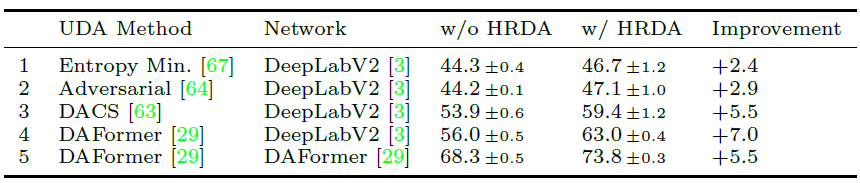
Influence of Resolution and Crop Size on UDA
HRDA 最一開始的假設是認為小的解析度會不好預測小物體,反之大的解析度會不好預測大物件,因此這一部分就是要驗這這個假設。
他們比較了 DAFormer 以及 HRDA 在 GTA5 Cityscapes 中調整 Crop Size 帶來的影響後發現,Crop Size 對於 UDA 與 Supervised Learning 相比更加重要。可以看到左邊的部分,當我們把 Crop Size 調低 4 倍,出來的結果會比沒有調整還要降低約 30 mIoU。
同時,這裡也可以觀察到當我們把解析度提升,無論是對 UDA 或是 Supervised Learning 都會有正面的影響。
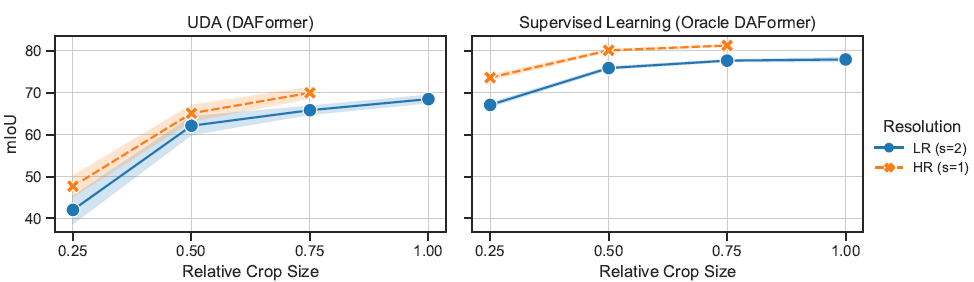
進一步去看每個 Class 的影響,可以觀察到:
- 比對 Row 1 與 Row 3,可以得到 Crop Size 越大,對所有 Class 的預測都有提升
- 比對 Row 1 與 Row 2,可以得到 Resolution 越大,小物件的預測更加精確,大物件則有降低的趨勢
- 將兩者結合後,在所有的 Class 的預測可以有更進一步的提升
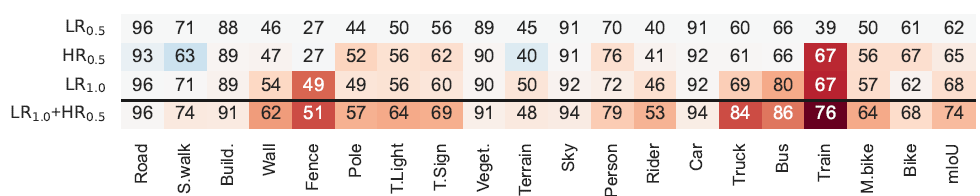
因此驗證了假設是正確的。
Crop Size Selection
針對 Context Crop Size 與 Detail Crop Size 做了詳細的比較如下表。
可以發現到:
- Context Crop Size 越大,得到的結果會更好
- Detail Crop Size 越大,得到的結果會更好
- 同樣的 Crop Size 下,再多搭配另一個 Resolution 都可以獲得更好的結果
- 不過相較之下,Low-Resolution 的有無對於最終結果的影響會是更大的

此外,HRDA 在 LR Crop 與 HR Crop 使用不同的 Resolution 是相當重要的。固定使用的 Context Crop 的情況下,如果採用低解析度的圖片,或是從低解析度 Upsample 到高解析度,得出來的效果都比起直接使用高解析度圖片還要差。
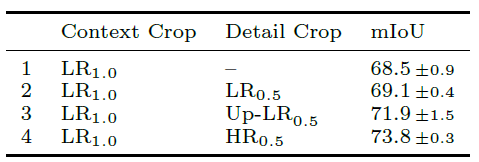
Memory Usage Comparison
HRDA 最一開始提到的問題就是使用 High Resolution 作為輸入會太過於耗費記憶體,因此這一部分會針對記憶體用量的部分去做討論。
註:這裡之所以是拿 作為 baseline 是因為 已經放不進 24GB 的顯卡了,並且 已經有比 DAFormer 更好的 performance。
比較之後可以發現到 HRDA 比起單純的 HR 可以有 3.8 mIoU 的成長,並且如果進一步把 Crop Size 縮小,即便只用了 HR 60% 的記憶體,但卻還是有 1.3 mIoU 的成長。
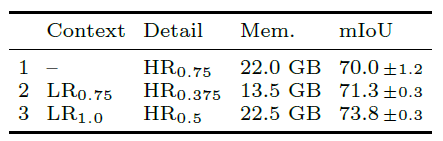
Ablation Study
在這一篇 paper 當中整體包含了幾個方法
- 混合 Context 與 Detail Crop
- 使用 Scale Attention 進一步產出合併結果
- Overlapping Detail 處理預測階段的 Detail Crop
- 加上 Detail Loss 進一步提升效能
針對這幾個部分同樣去研究對應的影響。
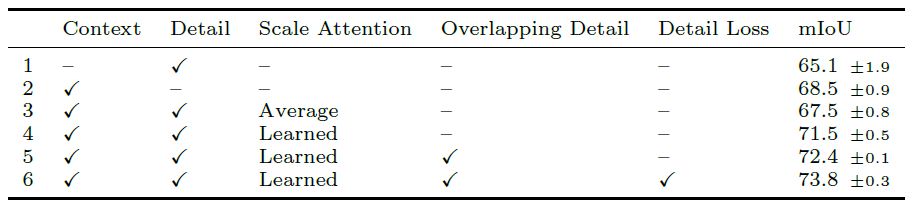
從上表可以觀察到
- Context 與 Detail 相較之下有更大的影響 (Row 1 & Row 2)
- 單純 Average Context 與 Detail 並不會有更好的 performance (Row 2 & Row 3)
- 採用 learned scale attention 對於效能提升大約 3.0 mIoU
- 採用 Overlapping Detail 可以進一步提升 0.9 mIoU
- 加上 Detail Loss 可以進一步提升 1.4 mIoU
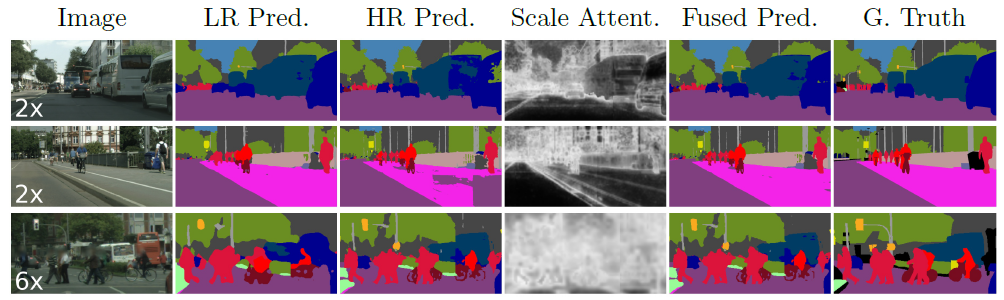
從圖片當中可以再次觀察到 LR 的結果在細節上會有缺失,HR 當中則對於大物件有較差的表現。
Contribution
- 研究了 Crop Size 對於 UDA performance 的影響
- 採用 multi-resolution fusion 搭配 scale attention 同時將 LR 與 HR 的優點帶走
- 在相同量級或甚至更小量級的記憶體使用量下,得到比 SOTA 更好的結果
- 提出一個能夠搭配許多 UDA Framework 的方法
- 提出新的 SOTA model